In conditionally automated driving (SAE Level 3), a human driver needs to quickly take over control of the vehicle when prompted to do so. A method for automatically recognizing that a driver has initiated the takeover process has now been developed.
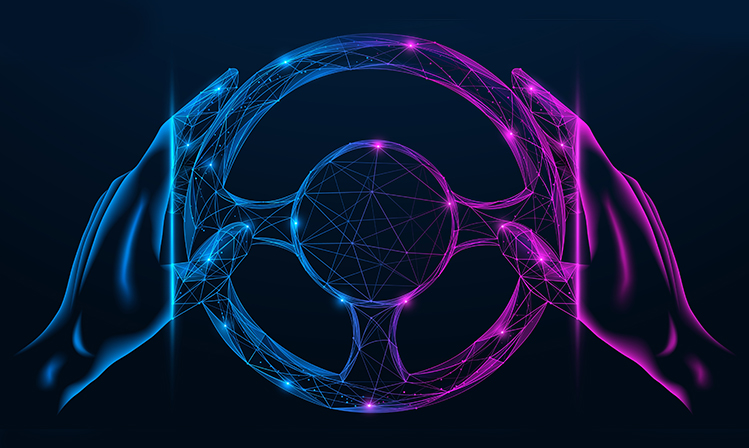
©︎ Ilya― stock.adobe.com/jp
Over 90% of traffic accidents are caused by human error. Research shows that automated driving promises to significantly reduce the number of accidents by reducing or eliminating human involvement. Fully automated driving under all road conditions is the ultimate goal, but partly automated driving, in which certain situations still require human driver intervention, is currently a more realistic compromise. Driver intervention needs to be prompted well in advance by the vehicle's human-machine interface, so that the human driver has enough time to prepare the takeover: eyes on the road, hands on the steering wheel, feet on the pedals. In studies assessing driver takeover behavior, labor intensive, manual annotation of video recordings is usually performed to identify the moment when the human driver reacts to a request to intervene.
Now, Takaaki Teshima and colleagues from Keio University have developed an automated method for determining the onset of a driver's preparatory action. Analyzing video footage of the driver's upper body, seat pressure and eye measurements, the method provides a binary signal indicating the beginning of a preparatory action. The method is expected to help verify that drivers begin preparing in time for manual takeover situations during automated drives.
"To our knowledge, there is no existing method for automatically identifying the onset of preparatory actions during takeovers," says Teshima.
Teshima and colleagues recruited 30 participants with driving experience for sessions in a driving simulator. The driving simulator had three displays, instrument panels, a steering wheel, and a gas and a brake pedal. The takeover situation was a road construction site, forcing the driver to manually change lanes ― a typical non-emergency scenario. The participants were prompted, by means of both a visual and an auditory signal, to change lanes 17 seconds before reaching the construction site, the time estimated for a safe takeover. The vehicle would automatically stop if the driver did not respond in time.
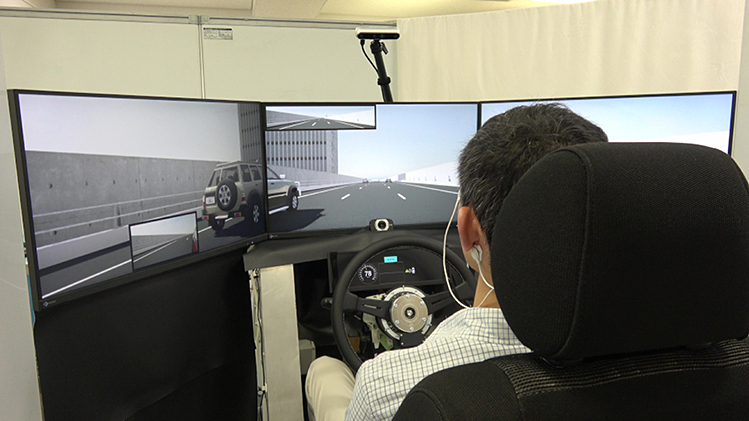
Fig. 6 from the paper
The driving simulator used for developing a method for the automatic determination of the onset of drivers' preparation for taking over control of an automatically driven car. (https://doi.org/10.1016/j.eswa.2024.123153) is licensed under CC-BY4.0
Video footage of the driver's upper body was converted into an animation of body key points to capture changes in the driver's posture and facial orientation. Changes in seat pressure were also monitored to help capture a shift in the driver's posture, and the driver's eye potential was measured by means of electrodes attached to the left and right temples and behind an ear. (This electrode setup measures so-called electrooculographic signals, which are generated when eyes move between left and right.)
The researchers developed a neural network model that took these upper-body, seat, and eye data as inputs. The model predicted whether the driver was performing a preparatory action with an accuracy of 93.88%. An algorithm was then applied to determine the onset of preparatory action based on the predicted probabilities, with a time error of 0.153 seconds relative to the actual onset.
Teshima and colleagues found that the electrooculographic measurements contributed little to the model's efficiency, but this could be due to the non-emergency nature of the simulation, and to the fact that the employed scenario featured low traffic density only.
The reported method enables the automatic determination of the point in time when a driver begins preparing to take over control of the vehicle, as a binary signal. Quoting the researchers, it "could serve to verify whether a request to intervene ... leads a driver to initiate a preparatory action within the expected time frame".
In the future, the researchers are planning to integrate the latest deep learning techniques for a real-time and vehicle-integrated approach for the next generation of automotive human-machine-interface systems.
Published online 26 September 2024
About the researcher
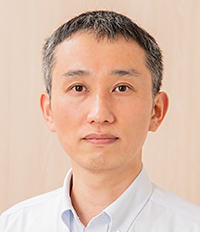
Takaaki Teshima ― Project Assistant Professor (Non-tenured)
Graduate School of System Design and Management
Takaaki Teshima graduated from Keio University in 2005 with a Bachelor in Environmental Information, and worked in the development of embedded software for around 10 years. He earned a Master of System Engineering degree, also from Keio University, in 2018. Teshima's research interests include driver behavior during automated driving, as well as his personal research on software visualization and model-driven development using the Unified Modeling Language (UML).
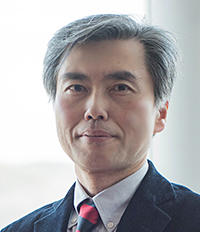
Hidekazu Nishimura ― Professor
Graduate School of System Design and Management
Hidekazu Nishimura received a Ph.D. in mechanical engineering from Keio University in 1990.
He was an Associated Professor at Chiba University from 1995 to 2007, a Visiting Researcher with the Delft University of Technology in the Netherlands in 2006, and a Visiting Associate Professor with the University of Virginia, Charlottesville in the US, in 2007. He is currently a Professor at the Keio University Graduate School of System Design and Management. His research interests include applying model-based systems engineering approach for various highly complex systems as well as designing an automated driving system.
Links
Reference
- Teshima, T., Niitsuma, M. & Nishimura, H. Determining the onset of driver's preparatory action for take-over in automated driving using multimodal data. Expert Syst. Appl. 246, 123153 (2024). | article