AI technology is increasingly being used in sports to analyze and improve the performance of athletes and teams. In particular, video analysis is proving to be an effective method for evaluating the performance of players and developing team tactics.
"In rugby, to analyze formation using ball and player position, it is necessary to detect play events such as scrums and lineouts," explains Yoshimitsu Aoki, a professor at the Faculty of Science and Technology at Keio University. "Until now, these tasks have been carried out by specialist analysts. The aim of my research is to automate these tasks using 'AI image technology.' Specifically, the ball and players are automatically detected from the video, and passages of play such as passes, kicks, and scrums are recognized based on such information."
Aoki and his colleagues are developing an innovative rugby video analysis system using deep learning technology. They are able to track players using an object detection method called 'Faster RCNN.' RCNN, or Regions with Convolutional Neural Networks, are improved version of the more conventional Convolutional Neural Networks (CNN) that allow for object detection in addition to image classification. "We then input rugby images as teacher data and performed additional learning using CNN," says Aoki. "Sequences of play are identified by giving time-series neural networks with extracted features such as player and ball position information."
As shown in the video, the ball and players are accurately detected and tracked, while their positions are mapped on the field. Using this method, passages of play can be automatically estimated and tagged so that a user can easily search for the desired sequence.
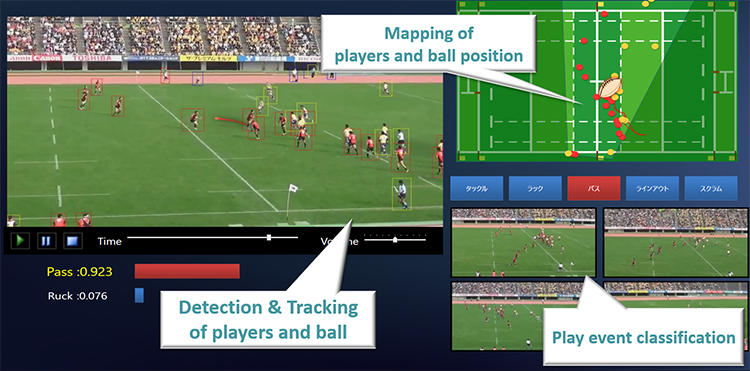
Rugby Video Analysis System : Automatic extraction of important scenes from video for supporting performance evaluation and planning team tactics © Aoki Media Sensing Lab., Keio University
"Using this system, statistical information from sports matches can be obtained rapidly. We are planning to apply this technology to other sports and action recognition issues."
Published online 24 December 2019
About the researcher
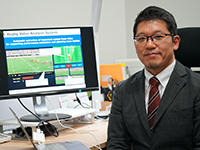
Yoshimitsu Aoki ― Professor
Department of Electronics and Electrical Engineering, Faculty of Science and TechnologyYoshimitsu Aoki received a B.E., M.E., and Doctorate in Applied Physics from Waseda University in 1996, 1998, 2001 respectively. He is currently a Professor at the Department of Electrical Engineering, Keio University. His research interests are in image processing, computer vision, pattern recognition, and artificial intelligence systems.
Links
Reference
- Kohei Hakozaki, Naoki Kato, Masamoto Tanabiki, Junko Furuyama, Yuji Sato, and Yoshimitsu Aoki, "Swimmer's stroke estimation using CNN and MultiLSTM", Journal of Signal Processing, Vol. 22, No. 4, pp. 219-222, July 2018. | article
- Christian Lanius, Daisuke Kobayashi, Kazushige Ouchi, and Yoshimitsu Aoki, "Single Image, Context Aware Action Estimation in Sports", 14th International Conference on Signal Image Technology and Internet Based Systems 2018. | article
- Shuto Horie, Yuji Sato, Junko Furuyama, Masamoto Tanabiki and Yoshimitsu Aoki, "Shot Detection in Racket Sport Video at the Frame Level Using A Recurrent Neural Network", 15th International Conference on Signal-Image Technology & Internet-Based Systems (SITIS) Sorrento, Italy, 2019, pp. 447-453. | article